文章信息
- 曾文婷, 韩志刚, 吴昊, 黎庆梅, 梁彩云, 徐理倩, 赵新华.
- Zeng Wenting, Han Zhigang, Wu Hao, Li Qingmei, Liang Caiyun, Xu Liqian, Zhao Xinhua
- 广州市2008-2017年注射吸毒人群HIV-1分子网络特征分析
- Analysis on characteristics of HIV-1 molecular network in injected drug users in Guangzhou, 2008-2017
- 中华流行病学杂志, 2021, 42(7): 1260-1265
- Chinese Journal of Epidemiology, 2021, 42(7): 1260-1265
- http://dx.doi.org/10.3760/cma.j.cn112338-20201209-01393
-
文章历史
收稿日期: 2020-12-09
2. 广州市疾病预防控制中心艾滋病预防控制部 510440
2. Department of HIV/AIDS Control and Prevention, Guangzhou Municipal Center for Disease Control and Prevention, Guangzhou, 510440, China
分子网络分析方法可从分子角度客观发现HIV-1感染者之间的可能传播关系[1-2],现已广泛应用于HIV相关研究[3]。常用的方法有成簇分析、连接分析和高危传播者分析[4]。当多个HIV-1感染者的病毒基因聚集成簇,提示他们之间存在直接或间接的关联[5],进行成簇分析可获取成簇人群的基本特征。而进一步构建分子网络则能了解成簇个体的连接程度,识别网络内中心性较大的节点。研究表明,对分子网络分析中发现的高风险传播者和高风险因素实施干预,可有效控制疾病传播[6-8]。
注射吸毒人群是HIV传播的高危人群之一。随着国家艾滋病免费抗病毒治疗、针具交换项目及美沙酮维持治疗等措施的实施[9],我国注射吸毒传播HIV得到有效控制[10]。近期有研究发现,相比于性传播人群,注射吸毒人群更容易出现HIV-1流行亚型改变及耐多药情况[11-13],是艾滋病防治工作的一个新挑战。本研究采用分子网络分析方法分析2008-2017年广州市注射吸毒人群HIV-1分子网络的分布特征,为该人群艾滋病防控提供参考依据。
对象与方法1. 样本来源:选取2008-2017年广州市新确证HIV-1感染者血清样本。纳入标准:①确证时现住址为广州市;②感染途径为注射吸毒传播。排除标准:血清样本容量 < 140 µl或未能获得目的基因序列片段的样本。从中国艾滋病综合防治信息系统获得样本的社会人口学资料。本研究通过广州市CDC伦理委员会审批(GZCDC伦理审查[项]2017030)。
2. 血清标本HIV RNA提取:采用QIAamp RNA Mini Kit试剂盒,按照说明书从血清中提取RNA,置于-80 ℃保存备用。
3. pol区基因扩增及测序:采用巢式PCR方法分两轮进行HIV-1 pol部分基因片段(HXB2:2 253~3 869)扩增,PCR扩增结果经琼脂糖凝胶电泳鉴定后送上海美吉生物医学科技有限公司测序。
4. 序列拼接与比对:使用Chromas 2.6.5软件检查序列质量,进行序列编辑、校正;使用Chromas Pro 2.1.3软件检查混合碱基并完成拼接;通过MEGA 7.0.26软件将拼接好的序列片段与Los Alamos HIV databases下载的参考序列进行比对和校正。
5. 序列亚型鉴定:利用Fast Tree 2.1.10软件通过最大似然法构建系统进化树。使用FigTree v1.4.3软件查看树形,根据样本序列与参考序列的拓扑关系判断样本的亚型。对于拓扑关系不明确或怀疑有重组的样本,使用HIV Database提供的在线工具重组识别软件RIP 3.0及在线数据库HIV circulating recombinant forms(CRFs),将获得的图谱与标准参考株图谱和最新流行重组模型进行对比。
6. HIV-1分子网络构建:由于样本时间跨度较大,以及本研究的主要目的是识别具有直接或间接传播关系的成员组成的网络,参考相关文献资料[14-16],以基因距离≤4.5%、分支支持度≥90%为阈值,使用Cluster Picker 1.2.3软件识别系统进化树中的分子簇。利用HyPhy 2.2.4软件中TN93模型计算成簇序列间的基因距离,根据最小基因距离准则鉴定簇内序列之间的关系[17-18],通过可视化软件Cytoscape 3.8.2构建分子网络。
7. 分子网络中心性度量定义:利用常用的网络度量包括程度中心性(degree centrality)、中介中心性(betweenness centrality)和亲近中心性(closeness centrality)进行分子网络中心性分析[19-20]。其中,程度中心性是节点的连接数之和的衡量指标,记为CD(ni),节点拥有越多连接则越可能占据有利位置,连接数≥3即定义为“高中心性”[21];中介中心性是对给定节点在其他两个节点之间最短路径上的频率的度量,记为CB(ni)衡量一个节点作为媒介者的能力,中介中心性越高,与其他节点之间的“桥梁”地位可能越重要;亲近中心性指给定节点与其他节点的距离之和的倒数,记为CC(ni),通常衡量该节点到网络中其他可到达节点的速度,亲近中心性越高,即与其他节点越接近,其传递信息方面就更加容易,因而更可能居于网络的中心。中介中心性和亲近中心性取值范围介于0~1之间,均以≥0.75定义为“高中心性”[22]。具有高程度中心性、高中介中心性和高亲近中心性特征的HIV-1感染者为“关键人物”。公式:
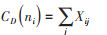
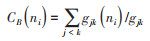

式中:Xij是0或1的数值,代表节点j是否与节点i有关系;gjk是节点j达到节点k的捷径数,gjk(ni)是节点j达到节点k的快捷方式数,g是此网络中的人数;d(ni,nj)代表ni与nj之间的距离。
8. 统计学分析:运用SPSS 20.0软件进行统计学分析,定量资料以x±s、定性资料以频数(构成比)表示,采用χ2检验或确切概率法进行成簇分析及分子网络中心性分析。双侧检验,以P < 0.05为差异有统计学意义。
结果1. 样本扩增结果:共收集793份血清样本,剔除未获得基因片段、序列质量不佳、混合碱基高于5%、条带过短及重复检测的序列,成功扩增pol区基因片段586条(73.9%,586/793)。
2. 样本人群社会人口学特征:共586条序列纳入本次分析,样本人群中,年龄(38.1±8.6)岁,年龄范围17~67岁,男性占89.2%(523/586),91.6%(537/586)为汉族,婚姻状况以未婚为主(61.4%,360/586),文化程度以初中及以下为主(85.1%,499/586),职业主要为家务及待业(56.1%,329/586),户籍主要为广东省(47.3%,277/586)及广西壮族自治区(广西)(25.1%,147/586),2008-2015年确证者占大多数(70.3%,412/586)。见表 1。
3. 成簇情况及其影响因素分析:序列亚型以CRF07_BC(48.1%,282/586)、CRF08_BC(22.7%,133/586)、CRF01_AE(22.7%,133/586)为主。共80个分子簇(每个簇至少包含2条序列),涉及273条序列,成簇率46.6%(273/586)。其中3个较大的分子簇序列分别由13条CRF07_BC、17条CRF07_BC和14条CRF55_01B亚型聚集形成。成簇样本分析结果显示,汉族(48.4%,260/537,260/537)、广东籍(52.7%,146/277)和CRF55_01B(93.3%,14/15的成簇比例高于未成簇样本。两组间的性别、年龄、婚姻状况、文化程度、职业和确证年份的差异无统计学意义。见表 1。
4. 分子网络中心性分析:对含至少3条序列的分子簇构建分子网络进行中心性分析,分子网络由187个节点和159条边构成,度值范围为1~7。度值≥3的节点占12.8%(24/187),其与网络中另外81个节点相关联(43.3%,81/187)。中心性分析结果显示,HIV-1感染者职业为家务/待业者在高程度中心性(19.0%,19/100)、高中介中心性(22.0%,22/100)和高亲近中心性(32.0%,32/100)的比例更高。未发现性别、年龄、婚姻状况、民族、文化程度、户籍和确证年份与分子网络中心性高低有统计学关联。见图 1、表 2。
![]() |
图 1 2008-2017年广州市注射吸毒人群HIV-1分子网络图 |
2008-2017年广州市注射吸毒人群HIV-1分子成簇率为46.6%,低于同期广州市MSM(90.3%)和异性性传播人群(58.5%)[23],提示注射吸毒人群在广州市传播和扩散HIV-1的危险性低于性传播人群,与我国艾滋病疫情已从注射吸毒传播为主转为主要经性传播的特点相符[24]。
成簇分析结果显示,本地户籍的注射吸毒人群更可能聚集成簇,可能原因是本地户籍的注射吸毒人群相比于非本地户籍者人际交往更广泛,从而促进了HIV-1传播。值得关注的是,既往研究显示广西籍注射吸毒人群是广州市艾滋病防治的重点人群[11],广西籍样本成簇率高(46.3%),提示需要对广西籍注射吸毒人群加强干预。另外,本研究发现CRF07_BC和CRF55_01B亚型更易聚成大簇,在注射吸毒人群中造成HIV-1传播和扩散的危险性高于其他亚型。而CRF07_BC和CRF55_01B在广州市性传播人群中也同样常见,且具有较高成簇率[23, 25],提示注射吸毒人群与性传播人群之间可能存在传播关联。有研究认为,导致注射吸毒人群感染HIV-1的更可能是同性性行为而非共用针具吸毒[26],结合该人群普遍存在高危性行为的特点[27-28],需进一步探讨注射吸毒人群和性传播人群的跨群传播关系。
利用多种中心性度量进行中心性分析能更明确“关键人物”在传播中的重要地位[29-30]。“关键人物”中心性越高,意味着其连接越多的HIV-1感染者。本研究结果显示虽然高度值的注射吸毒人群(“关键人物”)比例仅12.8%,但在分子网络中与另外81个节点关联了43.3%的HIV-1感染者,提示少数“关键人物”在HIV-1传播过程中发挥了重要作用,精准定位到这些“关键人物”并实施干预,将能更有效遏制艾滋病传播[8]。另外,本研究发现家务/待业者更可能在网络中占据中心位置。既往有研究认为,家务/待业的注射吸毒人群容易出现经济问题,增加了发生共用针具吸毒和商业性行为的可能性,从而提高了HIV-1感染风险[31-33]。而吸食毒品后个体社会功能削弱也将导致失业率提高,形成恶性循环[34]。提示我们需要从健康宣教、促进检测、抗病毒治疗、经济和社会支持等多方面共同入手,为注射吸毒人群提供支持。
分子网络分析结果可提示HIV-1感染者之间可能存在着传播关联,但需要进一步开展深入流行病学调查,结合行为学信息和分子信息才能更准确描述疾病传播关系,以实施精准干预。另外,本次研究纳入的为新报告病例而非新近感染病例,加上分析时间存在一定的滞后性,需要结合其他数据谨慎解读结果。
综上所述,广州市注射吸毒人群HIV-1分子成簇较复杂,HIV-1传播风险较高,应将具有本地及广西籍、家务/待业特征的注射吸毒人群作为重点干预目标,并针对其中的“关键人物”实施精准干预,降低该人群HIV-1感染率。
利益冲突 所有作者均声明不存在利益冲突
[1] |
de Oliveira T, Kharsany ABM, Gräf T, et al. Transmission networks and risk of HIV infection in KwaZulu-Natal, South Africa: a community-wide phylogenetic study[J]. Lancet HIV, 2017, 4(1): e41-50. DOI:10.1016/S2352-3018(16)30186-2 |
[2] |
中国疾病预防控制中心, 性病艾滋病预防控制中心. HIV传播网络监测和干预技术指南(试行)[DB/OL]. (2019-08)[2020-10-13]. http://iws365.com/pub/aws/pdf/HIVTechnicalGuide.pdf. National Center for AIDS/STD Control and Prevention, Chinese Center for Disease Control and Prevention. Technical guidelines for NETWORK monitoring and intervention of HIV Transmission (trial)[DB/OL]. (2019-08)[2020-10-13]. http://iws365.com/pub/aws/pdf/HIVTechnicalGuide.pdf. |
[3] |
甘梦泽, 冯毅, 邢辉. 基于分子网络方法研究HIV感染者传播特征的相关进展[J]. 中华流行病学杂志, 2019, 40(11): 1487-1491. Gan MZ, Feng Y, Xing H. Progress in research on the transmission characteristics of HIV-infected persons based on molecular network method[J]. Chin J Epidemiol, 2019, 40(11): 1487-1491. DOI:10.3760/cma.j.issn.0254-6450.2019.11.026 |
[4] |
黎裕雪云, 冯毅, 阮玉华, 等. HIV分子传播网络研究进展[J]. 中国艾滋病性病, 2016, 22(11): 940-942. Li YXY, Feng Y, Ruan YH, et al. Advance of HIV molecular transmission network[J]. Chin J AIDS STD, 2016, 22(11): 940-942. DOI:10.13419/j.cnki.aids.2016.11.29 |
[5] |
Wang KL, Yan HM, Liu YL, et al. Increasing prevalence of HIV and syphilis but decreasing rate of self-reported unprotected anal intercourse among men who had sex with men in Harbin, China: results of five consecutive surveys from 2006 to 2010[J]. Int J Epidemiol, 2012, 41(2): 423-432. DOI:10.1093/ije/dyr182 |
[6] |
Sabidussi G. The centrality index of a graph[J]. Psychometrika, 1966, 31(4): 581-603. DOI:10.1007/BF02289527 |
[7] |
Zhong L, Zhang QP, Li XM. Modeling the intervention of HIV transmission across intertwined key populations[J]. Sci Rep, 2018, 8: 2432. DOI:10.1038/s41598-018-20864-6 |
[8] |
Wang XC, Wu YS, Mao L, et al. Targeting HIV prevention based on molecular epidemiology among deeply sampled subnetworks of men who have sex with men[J]. Clin Infect Dis, 2015, 61(9): 1462-1468. DOI:10.1093/cid/civ526 |
[9] |
金晔, 卢洪洲. 吸毒感染艾滋病的主要方式及干预措施研究进展[J]. 中国艾滋病性病, 2020, 26(7): 794-796. Jin Y, Lu HZ. Overview on HIV infections among people who use drugs[J]. Chin J AIDS STD, 2020, 26(7): 794-796. DOI:10.13419/j.cnki.aids.2020.07.33 |
[10] |
吴尊友. 中国特色的艾滋病防治策略[J]. 中华疾病控制杂志, 2019, 23(8): 885-889. Wu ZY. HIV/AIDS prevention strategy with Chinese characteristics[J]. Chin J Dis Control Prev, 2019, 23(8): 885-889. DOI:10.16462/j.cnki.zhjbkz.2019.08.001 |
[11] |
赵宇腾, 韩志刚, 吴昊, 等. 广州市2008-2015年注射吸毒人群HIV-1亚型分布特征及变化趋势[J]. 中华流行病学杂志, 2019, 40(12): 1629-1633. Zhao YT, Han ZG, Wu H, et al. Characteristics and dynamics of HIV-1 subtype distribution among injected drug users in Guangzhou, 2008-2015[J]. Chin J Epidemiol, 2019, 40(12): 1629-1633. DOI:10.3760/cma.j.issn.0254-6450.2019.12.023 |
[12] |
徐理倩, 韩志刚, 张亚丽, 等. 广州市2008-2015年未接受抗病毒治疗的注射吸毒人群HIV-1感染者耐药分析[J]. 中华流行病学杂志, 2019, 40(2): 196-201. Xu LQ, Han ZG, Zhang YL, et al. Prevalence of antiretroviral drug resistance in treatment-naïve injecting drug users infected with HIV-1 in Guangzhou, 2008-2015[J]. Chin J Epidemiol, 2019, 40(2): 196-201. DOI:10.3760/cma.j.issn.0254-6450.2019.02.014 |
[13] |
董敖渤, 肖琳, 梁姝, 等. 凉山彝族自治州HIV/AIDS抗病毒治疗前HIV-1耐药情况及其影响因素分析[J]. 中华流行病学杂志, 2019, 40(6): 648-653. Dong AB, Xiao L, Liang S, et al. HIV-1 drug resistance and influencing factors among people living HIV/AIDS before antiretroviral therapy in Liangshan Yi Autonomous Prefecture[J]. Chin J Epidemiol, 2019, 40(6): 648-653. DOI:10.3760/cma.j.issn.0254-6450.2019.06.009 |
[14] |
Mbisa JL, Kirwan P, Tostevin A, et al. Determining the origins of human immunodeficiency virus type 1 drug-resistant minority variants in people who are recently infected using phylogenetic reconstruction[J]. Clin Infect Dis, 2019, 69(7): 1136-1143. DOI:10.1093/cid/ciy1048 |
[15] |
Sivay MV, Palumbo PJ, Zhang YF, et al. HIV drug resistance, phylogenetic analysis, and superinfection among men who have sex with men and transgender women in sub-Saharan Africa: HPTN 075[J]. Clin Infect Dis, 2020(8): ciaa1136. DOI:10.1093/cid/ciaa1136 |
[16] |
Rose R, Lamers SL, Dollar JJ, et al. Identifying transmission clusters with cluster picker and HIV-TRACE[J]. AIDS Res Hum Retroviruses, 2017, 33(3): 211-218. DOI:10.1089/AID.2016.0205 |
[17] |
Li XS, Xue YL, Lin Y, et al. Evolutionary dynamics and complicated genetic transmission network patterns of HIV-1 CRF01_AE among MSM in Shanghai, China[J]. Sci Rep, 2016, 6: 34729. DOI:10.1038/srep34729 |
[18] |
李诗韵, 戴洁, 孙鹏艳, 等. 昆明市2016-2018年男男性行为人群HIV-1分子网络特征分析[J]. 中华流行病学杂志, 2020, 41(7): 1121-1125. Li SY, Dai J, Sun PY, et al. Analysis on characteristics of HIV-1 molecular networks in men who have sex with men in Kunming, 2016-2018[J]. Chin J Epidemiol, 2020, 41(7): 1121-1125. DOI:10.3760/cma.j.cn112338-20190916-00676 |
[19] |
Carrington PJ, Scott J, Wasserman S. Models and methods in social network analysis[M]. New York: Cambridge University Press, 2005.
|
[20] |
罗家德. 社会网分析讲义[M]. 2版. 北京: 社会科学文献出版社, 2010. Luo JD. Social network analysis[M]. 2nd ed. Beijing: Social Sciences Academic Press, 2010. |
[21] |
de Arruda GF, Barbieri AL, Rodríguez PM, et al. Role of centrality for the identification of influential spreaders in complex networks[J]. Phys Rev E, 2014, 90(3): 032812. DOI:10.1103/PhysRevE.90.032812 |
[22] |
Little SJ, Pond SLK, Anderson CM, et al. Using HIV networks to inform real time prevention interventions[J]. PLoS One, 2014, 9(6): e98443. DOI:10.1371/journal.pone.0098443 |
[23] |
徐理倩. 广州市异性传播HIV亚型动态和传播簇研究[D]. 广州: 广东药科大学, 2020. DOI: 10.27690/d.cnki.ggdyk.2020.000049. Xu LQ. Study on subtype dynamics and transmission clusters of heterosexual transmission HIV in Guangzhou[D]. Guangzhou: Guangdong Pharmaceutical University, 2020. DOI: 10.27690/d.cnki.ggdyk.2020.000049. |
[24] |
汪宁, 钟平. 中国HIV分子流行病学30年[J]. 中华流行病学杂志, 2015, 36(6): 541-546. Wang N, Zhong P. Molecular epidemiology of HIV in China: 1985-2015[J]. Chin J Epidemiol, 2015, 36(6): 541-546. DOI:10.3760/cma.j.issn.0254-6450.2015.06.001 |
[25] |
韩志刚, 张亚丽, 吴昊, 等. 广州市2008-2015年男男性行为人群HIV-1亚型分布特征及变化趋势[J]. 中华流行病学杂志, 2018, 39(1): 67-71. Han ZG, Zhang YL, Wu H, et al. Characteristic and dynamic of HIV-1 subtype distribution in men who have sex with men in Guangzhou, 2008-2015[J]. Chin J Epidemiol, 2018, 39(1): 67-71. DOI:10.3760/cma.j.issn.0254-6450.2018.01.014 |
[26] |
Oster AM, Wertheim JO, Hernandez AL, et al. Using molecular HIV surveillance data to understand transmission between subpopulations in the United States[J]. J Acquir Immune Defic Syndr, 2015, 70(4): 444-451. DOI:10.1097/QAI.0000000000000809 |
[27] |
Paraskevis D, Nikolopoulos GK, Sypsa V, et al. Molecular investigation of HIV-1 cross-group transmissions during an outbreak among people who inject drugs (2011-2014) in Athens, Greece[J]. Infect Genet Evol, 2018, 62: 11-16. DOI:10.1016/j.meegid.2018.04.010 |
[28] |
Brookmeyer KA, Haderxhanaj LT, Hogben M, et al. Sexual risk behaviors and STDs among persons who inject drugs: A national study[J]. Prev Med, 2019, 126: 105779. DOI:10.1016/j.ypmed.2019.105779 |
[29] |
Mallik MK. An attempt to understand glioma stem cell biology through centrality analysis of a protein interaction network[J]. J Theor Biol, 2018, 438: 78-91. DOI:10.1016/j.jtbi.2017.11.001 |
[30] |
Karabekmez ME, Kirdar B. A novel topological centrality measure capturing biologically important proteins[J]. Mol Biosyst, 2016, 12(2): 666-673. DOI:10.1039/c5mb00732a |
[31] |
Paraskevis D, Nikolopoulos G, Fotiou A, et al. Economic recession and emergence of an HIV-1 outbreak among drug injectors in Athens metropolitan area: a longitudinal study[J]. PLoS One, 2013, 8(11): e78941. DOI:10.1371/journal.pone.0078941 |
[32] |
Temereanca A, Oprea C, Wertheim JO, et al. HIV transmission clusters among injecting drug users in Romania[J]. Rom Biotechnol Lett, 2017, 22(1): 12307-12315. |
[33] |
Milloy MJ, Kerr T, Buxton J, et al. Social and environmental predictors of plasma HIV RNA rebound among injection drug users treated with antiretroviral therapy[J]. J Acquir Immune Defic Syndr, 2012, 59(4): 393-399. DOI:10.1097/QAI.0b013e3182433288 |
[34] |
王娜, 刘效峰, 张正琪, 等. 上海市宝山区399名社区吸毒人员健康状况与社会支持特征分析[J]. 职业与健康, 2020, 36(4): 502-505, 510. Wang N, Liu XF, Zhang ZQ, et al. Analysis on health status and characteristics of social support in 399 community drug users in Baoshan District of Shanghai[J]. Occup Health, 2020, 36(4): 502-505, 510. DOI:10.13329/j.cnki.zyyjk.2020.0134 |