Article Information
- 廖立敏, 黄茜, 李建凤
- LIAO Li-min, HUANG Xi, LI Jian-feng
- 芳香醚类化合物13C NMR化学位移模拟
- Modeling of 13C NMR Chemical Shifts in Aromatic Ethers
- 波谱学杂志, 2016, 33(3): 368-377
- Chinese Journal of Magnetic Resonance, 2016, 33(3): 368-377
- http://dx.doi.org/10.11938/cjmr20160302
-
Article History
Received date: 2015-09-06
Revised date: 2016-07-09


DOI:10.11938/cjmr20160302
2. College of Chemistry and Chemical Engineering, Chongqing University, Chongqing 400044, China
2. 重庆大学 化学化工学院, 重庆 400044
The nuclear magnetic resonance (NMR) spectroscopy including hydrogen spectra and carbon spectra, is an intuitive and necessary method to identify chemical structures of organic compounds. 13C NMR is also widely used for the study of dynamic processes and balance of organic compounds. 13C NMR containing the quantum information associated directly with chemical properties of carbon nucleus can be used to resolve the structure of target compound[1-3]. However, the type of organic matter is very diverse, and solely relying instrument is difficult to fully obtain 13C NMR data. Therefore, predicting spectroscopic data of compounds with quantitative structure spectroscopy relationship (QSSR) method shows extremely wide prospect of application. The molecular local chemical microenvironment can be reflected on NMR spectrum. The 13C NMR simulation study was firstly done by Grant[4] and Lindeman[5] for alkanes. In recent years, with the rapid development of computer technology, an increasingly number of researchers engage in the study of13C NMR spectra simulation, and some ideal results have been achieved already[6-9]. In this study, the atomic electronegative space distance vector (AESDV) has been constructed based on three-dimensional structure of the molecule, and then used to characterize the microenvironment of equivalent carbon atoms of some aromatic ethers. The AESDV combined with the atomic hybridation state index (AHSI) is employed to establish carbon atom chemical shift prediction model through multivariate linear regression. The stability and predictive ability of the model is evaluated through internal and external testing methods. The model with satisfactory performance can be used for 13C NMR chemical shift prediction of this class of compounds.
1 Methods and Principles 1.1 Data sets9 aromatic ether compounds are selected as the research samples. The molecular structures are shown in Fig. 1. 13C chemical shifts of 46 carbon atoms in the samples are obtained from the literature[10] and given in the δ (Exp.) column of Table 1.
![]() |
Fig. 1 Structures of the 9 compounds |
Compound No. | Carbon atom No. | v1 | v2 | v3 | v4 | v5 | AHSI | δ (Exp.) | δ (Cal.) |
1 | 1 | 0.2481 | 3.7382 | 0.0000 | 2.5678 | 0.0000 | 1.6667 | 157.6 | 158.1 |
2 | 0.4941 | 4.9099 | 0.0000 | 0.1045 | 0.0000 | 1.6667 | 130.5 | 132.3 | |
3 | 6.6462 | 3.8321 | 0.0000 | 0.0078 | 0.0000 | 1.6667 | 126.5 | 123.5 | |
4 | 6.7602 | 3.8035 | 0.0000 | 0.0034 | 0.0000 | 1.6667 | 120.3 | 123.4 | |
5 | 6.7402 | 3.8101 | 0.0000 | 0.0069 | 0.0000 | 1.6667 | 126.5 | 123.4 | |
6 | 6.7368 | 3.7800 | 0.0000 | 0.0817 | 0.0000 | 1.6667 | 110.2 | 124.1 | |
7 | 17.7340 | 0.1135 | 0.0000 | 2.1744 | 0.0000 | 1.2500 | 55.3 | 56.3 | |
2 | 1 | 0.1939 | 3.5965 | 0.0606 | 2.7583 | 0.0000 | 1.6667 | 153.3 | 160.5 |
2 | 0.1334 | 3.5245 | 1.5030 | 0.3324 | 0.0000 | 1.6667 | 139.9 | 143.0 | |
3 | 6.4508 | 3.7389 | 0.0672 | 0.0589 | 0.0000 | 1.6667 | 125.8 | 125.0 | |
4 | 6.7284 | 3.8125 | 0.0056 | 0.0086 | 0.0000 | 1.6667 | 120.9 | 123.4 | |
5 | 6.6782 | 3.8132 | 0.0026 | 0.0094 | 0.0000 | 1.6667 | 134.5 | 123.5 | |
6 | 6.6933 | 3.7681 | 0.0052 | 0.0878 | 0.0000 | 1.6667 | 114.3 | 124.4 | |
7 | 17.7037 | 0.1104 | 0.0028 | 2.141 | 0.0000 | 1.2500 | 57.4 | 56.2 | |
3 | 1 | 0.3041 | 3.7227 | 0.0000 | 2.5778 | 0.0000 | 1.6667 | 159.8 | 158.2 |
2 | 6.7440 | 3.7639 | 0.0000 | 0.0807 | 0.0000 | 1.6667 | 114.1 | 124.2 | |
3 | 6.7323 | 3.8009 | 0.0000 | 0.0069 | 0.0000 | 1.6667 | 129.6 | 123.5 | |
4 | 6.7698 | 3.7836 | 0.0000 | 0.0035 | 0.0000 | 1.6667 | 120.7 | 123.5 | |
5 | 17.7305 | 0.1107 | 0.0000 | 1.3887 | 0.0000 | 1.2500 | 54.7 | 51.9 | |
4 | 1 | 0.3040 | 3.7311 | 0.0000 | 2.5745 | 0.0000 | 1.6667 | 158.4 | 158.1 |
2 | 6.7408 | 3.7806 | 0.0000 | 0.0805 | 0.0000 | 1.6667 | 114.2 | 124.1 | |
3 | 6.6580 | 3.8281 | 0.0000 | 0.0069 | 0.0000 | 1.6667 | 130.4 | 123.5 | |
4 | 0.5906 | 4.9735 | 0.0000 | 0.0034 | 0.0000 | 1.6667 | 129.6 | 130.8 | |
5 | 17.7686 | 0.1108 | 0.0000 | 2.1781 | 0.0000 | 1.2500 | 55.2 | 56.3 | |
5 | 1 | 0.3002 | 3.7188 | 0.0000 | 1.6595 | 0.0022 | 1.6667 | 158.8 | 153.0 |
2 | 6.7200 | 3.7730 | 0.0000 | 0.0518 | 0.0045 | 1.6667 | 115.8 | 124.0 | |
3 | 6.5987 | 3.7788 | 0.0000 | 0.0044 | 0.0482 | 1.6667 | 129.9 | 123.2 | |
4 | 0.2349 | 3.7301 | 0.0000 | 0.0022 | 0.7433 | 1.6667 | 125.4 | 131.1 | |
5 | 17.7362 | 0.1107 | 0.0000 | 2.1678 | 0.0002 | 1.2500 | 54.6 | 56.3 | |
6 | 1 | 2.8592 | 3.6976 | 0.0000 | 0.0392 | 0.0000 | 1.6667 | 164.7 | 156.3 |
2 | 6.6493 | 3.7740 | 0.0000 | 0.0280 | 0.0000 | 1.6667 | 114.5 | 124.1 | |
3 | 0.1890 | 3.8298 | 0.0000 | 0.0029 | 0.0000 | 1.6667 | 130.5 | 142.9 | |
4 | 1.4459 | 5.0118 | 0.0000 | 0.0009 | 0.0000 | 1.6667 | 130.2 | 127.9 | |
5 | 17.7444 | 0.1106 | 0.0000 | 2.1589 | 0.0000 | 1.2500 | 56.1 | 56.2 | |
7 | 1 | 2.6769 | 3.7889 | 0.0000 | 0.0319 | 0.0000 | 1.6667 | 157.1 | 136.0 |
2 | 0.3680 | 4.9368 | 0.0000 | 0.1173 | 0.0000 | 1.6667 | 130.2 | 132.5 | |
3 | 0.1828 | 3.8278 | 0.0000 | 0.0077 | 0.0000 | 1.6667 | 128.5 | 142.9 | |
4 | 6.6289 | 3.8224 | 0.0000 | 0.0013 | 0.0000 | 1.6667 | 123.5 | 123.6 | |
8 | 1 | 0.1977 | 3.7854 | 0.0000 | 2.4755 | 0.0021 | 1.6667 | 156.6 | 157.3 |
2 | 0.4923 | 4.9395 | 0.0000 | 0.0928 | 0.0041 | 1.6667 | 133.6 | 131.9 | |
3 | 6.5341 | 3.8039 | 0.0000 | 0.0072 | 0.4451 | 1.6667 | 129.2 | 116.5 | |
4 | 0.2331 | 3.7708 | 0.0000 | 0.0035 | 0.6808 | 1.6667 | 129.2 | 131.8 | |
9 | 1 | 0.1968 | 3.7685 | 0.0027 | 2.5033 | 0.0000 | 1.6667 | 162.8 | 157.6 |
2 | 0.4900 | 4.9415 | 0.0055 | 0.0992 | 0.0000 | 1.6667 | 133.2 | 131.9 | |
3 | 6.4152 | 3.7668 | 0.0660 | 0.0564 | 0.0000 | 1.6667 | 125.2 | 124.8 | |
4 | 0.2285 | 3.6769 | 1.4740 | 0.2336 | 0.0000 | 1.6667 | 144.2 | 140.8 |
It is well known that the NMR chemical shifts are influenced by many factors, and the most important one is the local chemical microenvironment. Chemical microenvironment is associated with the distribution of electron cloud, which is associated with the kind and the number of atoms or groups bonded to the target atom. For most organic molecules, there are mainly several atoms including H, C, N, P, O, S, F, Cl, Br and I. They can be classified as 5 types of atoms according to the families of the periodic table of elements. It is shown that the chemical microenvironment is related closely to atomic electronegativity and distance. Considering atomic type, element electronegativity and space distance between atoms, a new vector called AESDV is developed to characterize the local chemical microenvironment. In AESDV, each atomic type is denoted by k (k=1~5), which means that there are k types of atoms linking to the examined atom. The 5 elements in the AESDV for the chosen carbon atom are defined as Eq. (1):
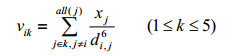
here, i represents the examined atom, j represents all atoms belonging to atomic type k, x is the atomic relative electronegativity, being xA=XA/XC, based on Pauling’s scale, defined as the ratio of atomic electronegativity to that of the carbon atom (2.55). For example, the relative electronegativity of atoms carbon and oxygen are 2.55/2.55=1.000 0 and 3.44/2.55=1.349 0, which are taken from the literature[11, 12]. In literature[13], di, j represents the ratio between the sum of the shortest bond length between atoms i and j and the C–C single bond distance [1.540×(0.1 nm) (Å)]. In this paper, di, j is improved in some degree, where di, j represents the relative distance between the ith and jth atoms, calculated as the ratio of the space distance to the single C–C bond length. The interaction effect between atoms i and j is in proportion to sixth power of relative distance between 5 different atoms and target atoms, so the denominator in Eq. (1) is raised to the sixth power. The 5 elements in the AESDV are noted as vH, vC, vN, vO and vX, shorted as v1, v2, v3, v4 and v5, respectively.
Here, the compound No. 2 is chosen as an example to illustrate the calculation process of the variables. Its original spatial structure is auto generated by software Chemoffice 2006, and then optimized preliminarily by using semi-empirical quantum chemistry soft MOPAC in Chem 3D at AM1 level (energy cut-off value: 0.001 kJ/mol). The optimized structure, space coordinates and relative electronegativity of all the atoms of compound No. 2 are obtained and shown in Fig. 2 and Table 2.
![]() |
Fig. 2 3D structure of compound No.2 |
No | Atom | x | X | Y | Z |
1 | C | 1.0000 | -0.7090 | 0.2035 | 0.0132 |
2 | C | 1.0000 | -0.7079 | -1.2153 | 0.0111 |
3 | C | 1.0000 | -1.9293 | -1.9118 | 0.0056 |
4 | C | 1.0000 | -3.1307 | -1.2138 | 0.0000 |
5 | C | 1.0000 | -3.1307 | 0.1827 | 0.0000 |
6 | C | 1.0000 | -1.9348 | 0.8907 | 0.0082 |
7 | C | 1.0000 | 0.5150 | 2.2473 | 0.0182 |
8 | N | 1.1922 | 0.5564 | -1.9879 | 0.0146 |
9 | O | 1.3490 | 0.5147 | 0.8207 | 0.0231 |
10 | O | 1.3490 | 1.6083 | -1.4119 | -0.0172 |
11 | O | 1.3490 | 0.4895 | -3.1917 | 0.0471 |
12 | H | 0.8627 | -4.0866 | 0.7301 | -0.0059 |
13 | H | 0.8627 | -4.0821 | -1.7658 | -0.0044 |
14 | H | 0.8627 | -1.9250 | -3.0167 | 0.0061 |
15 | H | 0.8627 | -1.9521 | 1.9900 | 0.0099 |
16 | H | 0.8627 | 1.6082 | 2.4945 | 0.0220 |
17 | H | 0.8627 | 0.0227 | 2.6390 | -0.9046 |
18 | H | 0.8627 | 0.0156 | 2.6439 | 0.9355 |
Here the v3 is calculated as an example. v3 is the effect item of the group IVA atoms on the target carbon atom (i.e., No.2). There is only one IVA atom (i.e., No.8) in the molecular structure. Firstly, the space distance (δ) between the atoms No.2 and No.8 should be calculated according to their spatial location coordinates d ={[0.556 4-(-0.707 9)]2+[-1.987 9-(-1.215 3)]2+(0.014 6-0.011 1)2}1/2=1.481 7×(0.1 nm)=1.481 7 Å, the relative distance d2, 8 =1.481 7/1.540=0.962 1. The relative electronegativity of atom No.8 is 1.192 2. v3of the atom No.2 can be calculated as: v3=1.192 2/0.962 16=1.503 0. Accordingly, v1, v2, v3, v4 and v5 of all target carbon atoms in the samples can be calculated as the same method and are listed in Table 1.
1.3 Atomic hybridization state indexIt is well known that chemical shifts are also influenced by the hybridization state of atoms. The atomic hybridization state index (AHSI) descriptor is introduced to characterize the hybridization state of the examined atom. The atomic AHSI is calculated by using the literature[13] method [Eq. (2)].

where, mi is the number of electrons in valence shell of the target atom i; ni the principal quantum number; δ(σ+π)i is the number of total electrons forming σ and π bonds; δ(σ)i is the number of only electrons forming σ bonds. The AHSI values of sp, sp2 and sp3 carbons are 2.500 0, 1.666 7 and 1.250 0, respectively. The AHSI values of all target carbon atoms in the samples are given in AHSI column in Table 1.
1.4 Evaluation of the methodFirstly, multiple linear regression (MLR) is employed to construct the model. And then the leave-one-out procedure (LOO) is used for internal cross-validation of the mode, and the specific process can reference the literature[14]. The correlation coefficient (RCV) and the standard deviation (SDCV) obtained in the internal cross-validation are adopted to evaluate the stability of the model. To illustrate the predictive power of the model, the samples are randomly divided into 2 groups. One group of the samples will be modeled as a training set, another group as a test set to test the model. The predictive power of the model is evaluated by using external forecast correlation coefficient (Rtest) and standard deviation (SDtest)[15].Rtest and SDtest are respectively calculated as Eq. (3) and Eq. (4).
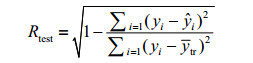
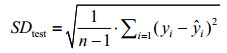
In Eq. (3) or Eq. (4), both yi and
The AESDV and AHSI descriptors (listed in Table 1) considered as the independent variables and the chemical shifts (CS) of 46 target carbon atoms of the 9 samples as the dependent variables, a MLR model [Eq. (5)] is constructed through the MLR method.

Model fitting: N=46, n=6, R1=0.964, SD1=8.673, F1=84.545;LOO cross-validation: RCV1=0.948, SDCV1=10.362, FCV1=57.282.
N is the regression points, n is the number of variables, R is the multiple correlation coefficient, SD is the standard deviation of estimate, F is the Fischer test value; RCV is the correlation coefficient of cross-validation, SDCV is the standard deviation of cross-validation, FCV is Fischer test value for the cross-validation.
The model complies with the empirical rule of N/n > 5. The R and RCV of the established model are desirable ( > 0.90), which indicates fine stability and predictability of the model. Another indicator used to evaluate the quality of model is the SD. The model is good and prediction accuracy is acceptable when the ratio of the SD to the value range is less than 10%[16]. The SD and SDCV of the model are 8.673 and 10.362, and the chemical shift value range of target carbon atoms in the samples between the maximum (164.7) and the minimum (54.6) values is 110.1 (164.7-54.6=110.1). The ratios of the SD1 and SDCV1 to 110.1 are 7.92% and 8.46%, respectively. They are all less than 10%, which indicate acceptable accuracy of the model prediction.
2.2 Model validation7 carbon atoms of the compound No.1 are randomly selected as the test set 1 to evaluate the model, and the 39 carbon atoms of the remaining 8 compounds assemble as the training set 1 to build up the model. The MLR model is constructed as Eq. (6).

Model fitting: N=39, n=6, R2=0.961, SD2=9.184, F2=63.833; LOO cross-validation: RCV2=0.942, SDCV2=11.118, FCV2=41.866; External forecast: Rtest1=0.979, SDtest1=6.400.
9 carbon atoms of the compounds No.3 and No.7 are randomly selected as the test set 2 to evaluate the model, and the 37 carbon atoms of the remaining 7 compounds assemble as the training set 2 to build up the model. The MLR model is constructed as Eq. (7).

Model fitting: N=37, n=6, R3=0.968, SD3=8.389, F3=74.827; LOO cross-validation: RCV3=0.942, SDCV3=11.280, FCV3=39.149; External forecast: Rtest2=0.939, SDtest2=10.162.
The R, RCV and Rtest of the two models are desirable ( > 0.90), indicating good predictive ability and strong stability of the two models. The chemical shift value range of target carbon atoms in the test set 1 between the maximum value (157.6) and the minimum value (55.3) is 102.3. The ratio of the SDtest1 to 102.3 is 6.26%. The chemical shift value range of target carbon atoms in the test set 2 between the maximum value (159.8) and the minimum value (54.7) is 105.1. The ratio of the SDtest2 to 105.1 is 9.67%. They are all less than 10%, which indicates acceptable accuracy of the model prediction. All the results show that the models constructed with the method introduced above have satisfactory performance.
2.3 Calculation results and errorsThe chemical shifts of 46 target carbon atoms of the 9 samples are calculated by the MLR model [Eq. (5)] and the results are listed in Table 1. Fig. 3 presents a plot of the experimented chemical shift values versus calculated ones, and Fig. 4 presents the residual distribution of calculated results. Fig. 3 shows that most of the sample points are close to the 45°diagonal line, indicating the good predictive ability and the small prediction errors of the model. As can be seen in Fig. 4, the range of“±2SD”of MLR model [Eq. (5)] is set as the standard and most of the sample points are not beyond this range, which shows the satisfactory simulation results of the model. The simulation errors of some few target carbon atoms directly connected with an oxygen atom or a chlorine atom are slightly larger. The oxygen atom or the chlorine atom may have affected the shielding effect of the extra-nuclear electron cloud of the target atoms. Chemical microenvironment of these target carbon atoms is not expressed absolutely, which may be the reason of the somewhat larger chemical shift errors of the target carbon atoms. The results indicate that the AESDV should be further improved in the expression of atomic special microenvironment.
![]() |
Fig. 3 Scatter plot of chemical shift estimated values vs. experimental values |
![]() |
Fig. 4 Residual distribution of calculated chemical shifts |
The NMR chemical shifts are mainly influenced by local chemical microenvironment and hybridization state of atoms. The AESDV can well reflect the different effects produced by other atoms on the target atom. The AHSI can effectively represent atom hybridization state. The model constructed in this study can accurately predict the 13C NMR chemical shifts of aromatic ether molecules, and the results of internal cross-validation are satisfactory. The compounds are randomly divided into 2 sets and the test set is used to evaluate the model, which makes the results with higher confidence. The results show that the method can accurately simulate the 13C NMR chemical shifts of aromatic ether compounds. This research has a certain theoretical significance and application value. But there remains a small number of carbon atoms whose chemical shifts simulation errors are slightly large, the method therefore needs further study and improvement.
[1] | 宋爱华, 张延峰, 沙沂, 等. 富马酸替诺福韦二吡呋酯波谱数据与结构确证[J]. 波谱学杂志 , 2015, 32 (3) : 542-550 Song Ai-hua, Zhang Yan-feng, Sha Yi, et al. Spectral analysis and structural elucidation of tenofovir disoproxil fumarate[J]. Chinese J Magn Reson , 2015, 32 (3) : 542-550 |
[2] | 吴春丽, 李杰明, 魏会杰, 等. 阿托伐他汀内酯的波谱学数据与结构确证[J]. 波谱学杂志 , 2014, 31 (2) : 268-277 Wu Chun-li, Li Jie-ming, Wei Hui-jie, et al. Spectral analysis and structural elucidation of atorvastatin lactone[J]. Chinese J Magn Reson , 2014, 31 (2) : 268-277 |
[3] | 杨晓艳, 吴香玉, 安艳捧, 等. 吲哚丙酮酸的酮-烯醇互变异构化的NMR研究[J]. 波谱学杂志 , 2014, 31 (1) : 81-90 Yang Xiao-yan, Wu Xiang-yu, An Yan-peng, et al. An NMR study on keto-enol tautomerism of indole-3-pyruvic acid[J]. Chinese J Magn Reson , 2014, 31 (1) : 81-90 |
[4] | Grant D M, Paul E G. Carbon-13 magnetic resonance Ⅱ. chemical shift data for the alkanes[J]. J A Chem Soc , 1964, 86 : 2984-2990 DOI:10.1021/ja01069a004 |
[5] | Lindeman L P, Adams J Q. Carbon-13 nuclear magnetic shifts for the resonance spectrometry[J]. Anal Chem , 1971, 43 (10) : 1245-1252 DOI:10.1021/ac60304a002 |
[6] | Tong J, Chen Y, Liu S, et al. Estimation and prediction of 13C NMR chemical shifts of carbon atoms in both alcohols and thiols[J]. J Struct Chem , 2012, 53 (6) : 1075-1080 DOI:10.1134/S002247661206008X |
[7] | Meiler J, Meusinger R, Will M. Fast determination of 13C NMR chemical shifts using artificial neural networks[J]. J Chem Inf Comput Sci , 2000, 40 (5) : 1169-1176 DOI:10.1021/ci000021c |
[8] | 田菲菲, 李志良, 吴世容, 等. 原子电性作用矢量用于呋喃糖核磁共振碳谱化学位移计算[J]. 波谱学杂志 , 2006, 23 (3) : 355-359 Tian Fei-fei, Li Zhi-liang, Wu Shi-rong, et al. Calculation of chemical shift in 13C NMR for furanoses[J]. Chinese J Magn Reson , 2006, 23 (3) : 355-359 |
[9] | 邓婕, 梅虎, 周鹏, 等. 原小檗属类生物碱13C核磁共振化学位移的模拟[J]. 波谱学杂志 , 2007, 24 (2) : 211-216 Deng Jie, Mei Hu, Zhou Peng, et al. Modeling quantitative structure-spectrum relationship of 13C NMR chemical shifts of protoberberine alkaloids[J]. Chinese J Magn Reson , 2007, 24 (2) : 211-216 |
[10] | 于德泉, 杨峻山. 分析化学手册[M].第二版. 北京: 化学工业出版社, 1999 : 575 Yu De-quan, Yang Jun-shan. Handbook of Analytical Chemistry[M].2nd ed. Beijing: Chemical Industry Press, 1999 : 575 |
[11] | AylwardG H, FindlayT J. SI化学数据手册[M].第二版. 北京: 高等教育出版社, 1985 : 94 Aylward G H, Findlay T J. SI Chemical Data[M].(2nd ed). Beijing: High Education Press, 1985 : 94 |
[12] | 张寒琦, 陈铮, 林英杰, 等. 实用化学手册[M]. 北京: 科学出版社, 2001 : 682 -723 Zhang Han-qi, Chen Zheng, Lin Ying-jie, et al. Handbook of Chemical Data[M]. Beijing: Science Press, 2001 : 682 -723 |
[13] | 周鹏, 周原, 梅虎, 等. Atomic electronegativity interaction vector and atomic hybridation state Index for spectroscopic smiulation of 13C muclear magnetic resonance of Amino acids[J]. Chin J Anal Chem , 2006, 34 (2) : 200-204 Zhou Peng, Zhou Yuan, Mei Hu, et al. Atomic electronegativity interaction vector and atomic hybridation state Index for spectroscopic smiulation of 13C muclear magnetic resonance of Amino acids[J]. Chin J Anal Chem , 2006, 34 (2) : 200-204 DOI:10.1016/S1872-2040(06)60013-3 |
[14] | 李波, 曾晖, 周鹏, 等. 三维原子场全息作用矢量用于7位取代20(S)-喜树碱衍生物的抗肿瘤活性定量构效关系研究[J]. 精细化工 , 2006, 23 (1) : 41-46 Li Bo, Zeng Hui, Zhou Peng, et al. QSAR studies of 7-substituted 20(S)-camptothecin analogues with antitumor activity using three-dmiensional holographic vector of atomic interaction field (3D-HoVAIF)[J]. Fine Chemicals , 2006, 23 (1) : 41-46 |
[15] | Golbraikh A, Tropsha A. Beware of q2![J]. J Mol Graph Model , 2002, 20 (4) : 269-276 DOI:10.1016/S1093-3263(01)00123-1 |
[16] | Sung-sau S, Karplus M. A comparative study of ligand-receptor complex binding affinity prediction methods based on glycogen phosphorylase inhibitors[J]. J Compu Aided Mol Des , 1999, 13 (3) : 243-258 DOI:10.1023/A:1008073215919 |